Introduction
Cardiovascular disease (CVD) remains the leading cause of mortality worldwide, necessitating continuous advancements in risk assessment and prevention strategies. Early and accurate identification of individuals at risk is crucial for implementing timely interventions and reducing morbidity and mortality rates. This necessity has driven substantial progress in cardiovascular risk stratification, a method that categorizes individuals based on their likelihood of developing cardiovascular events such as heart attacks or strokes. Among the many tools available, the cardiac risk index and various cardiac risk stratification models have evolved significantly, integrating novel biomarkers, genetic data, and advanced imaging techniques to enhance predictive accuracy.
You may also like: 5 Modern Treatments for Heart Disease: Advancements in Cardiac Care
Over the past decade, breakthroughs in artificial intelligence (AI), machine learning, and precision medicine have transformed the landscape of cardiac risk assessment. These innovations provide a more personalized approach to prevention, moving beyond traditional risk factors like cholesterol levels, hypertension, and smoking history. Instead, newer models incorporate individualized data, offering more precise risk evaluations and targeted interventions. This article explores the latest advancements in cardiovascular risk stratification, examining the evolving methodologies, emerging biomarkers, and the role of digital health in reshaping preventive cardiology.
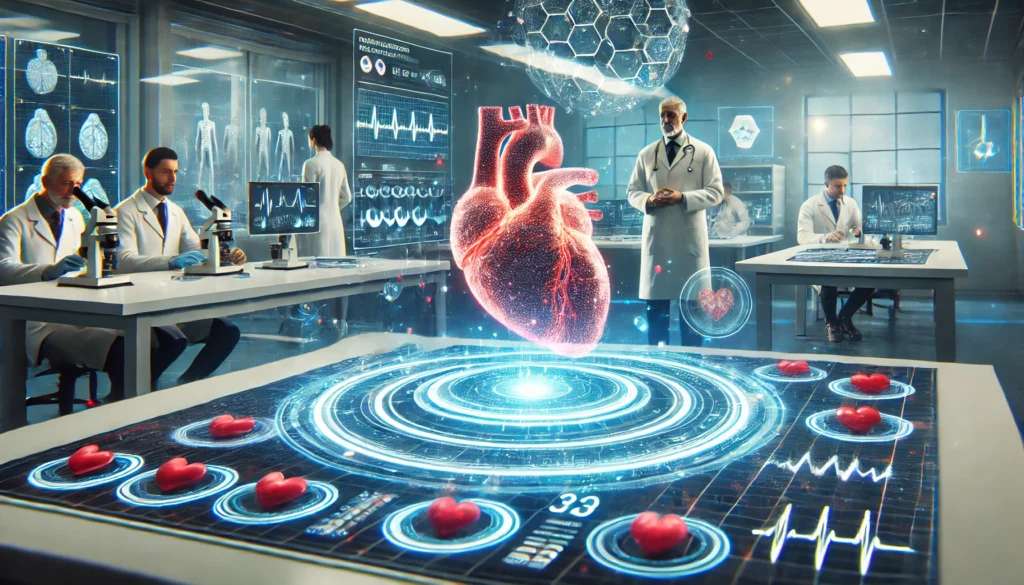
The Evolution of Cardiovascular Risk Stratification
Historically, cardiovascular risk assessment relied on simplistic models that estimated an individual’s likelihood of developing CVD based on broad population-based data. The Framingham Risk Score, one of the earliest models, provided a foundation for cardiovascular risk stratification by incorporating factors such as age, blood pressure, cholesterol levels, and smoking status. While these models offered valuable insights, they lacked precision when applied to diverse populations with varying genetic backgrounds and lifestyle factors.
Over time, refinements to risk prediction models have led to more comprehensive assessments. The introduction of the Atherosclerotic Cardiovascular Disease (ASCVD) Risk Calculator expanded upon previous models by integrating additional factors such as diabetes status and ethnicity. Despite these improvements, traditional models still faced limitations in accurately predicting cardiovascular events in certain populations. This gap in precision has driven the development of more sophisticated approaches that incorporate biomarkers, imaging techniques, and machine learning algorithms.
Recent advancements in cardiovascular risk stratification leverage big data and personalized medicine, allowing for a more tailored approach to risk assessment. For instance, polygenic risk scores (PRS) have emerged as a valuable tool in identifying individuals with a genetic predisposition to CVD, complementing traditional risk models. Additionally, inflammatory markers like high-sensitivity C-reactive protein (hs-CRP) and novel lipid biomarkers have provided deeper insights into atherosclerotic processes, enhancing risk prediction capabilities.
The Cardiac Risk Index: Refining Predictive Accuracy
The cardiac risk index remains a pivotal tool in evaluating the likelihood of adverse cardiac events, particularly in patients undergoing surgical procedures. Initially developed to assess perioperative cardiac risk, the index has undergone several refinements to enhance its predictive power. The Revised Cardiac Risk Index (RCRI) introduced additional variables such as renal dysfunction and diabetes mellitus, improving its accuracy in identifying high-risk individuals.
While the RCRI remains widely used, its limitations have prompted the development of more nuanced models. The American College of Cardiology (ACC) and the American Heart Association (AHA) have advocated for the integration of additional clinical and biochemical markers to refine risk stratification. For example, the inclusion of N-terminal pro-brain natriuretic peptide (NT-proBNP) levels has demonstrated superior predictive value in assessing perioperative cardiac risk.
Emerging research suggests that incorporating imaging-based assessments, such as coronary artery calcium (CAC) scoring and cardiac magnetic resonance (CMR) imaging, can further enhance the accuracy of the cardiac risk index. CAC scoring, in particular, has gained widespread recognition for its ability to quantify subclinical atherosclerosis, providing valuable prognostic information beyond traditional risk factors. As these imaging modalities become more accessible, their integration into routine cardiac risk assessment may revolutionize preventive cardiology.
Advanced Imaging Techniques in Cardiac Risk Stratification
The evolution of imaging technology has significantly contributed to the advancement of cardiovascular risk stratification. Traditional diagnostic tools like electrocardiograms (ECGs) and stress tests, while useful, often lack the sensitivity needed to detect early-stage cardiovascular disease. In contrast, contemporary imaging modalities offer detailed visualizations of vascular and myocardial health, enabling more precise risk assessment.
One of the most transformative imaging techniques is coronary computed tomography angiography (CCTA), which provides a non-invasive method to evaluate coronary artery disease (CAD). By detecting coronary plaque burden and arterial stenosis, CCTA allows for early intervention in high-risk individuals. Additionally, positron emission tomography (PET) imaging has emerged as a valuable tool in assessing myocardial inflammation and perfusion abnormalities, further refining risk prediction models.
Another promising advancement is the utilization of artificial intelligence in image analysis. AI-powered algorithms can analyze large datasets of imaging scans, identifying subtle abnormalities that may be overlooked by traditional interpretation methods. This technology enhances diagnostic accuracy and streamlines clinical decision-making, ultimately improving patient outcomes. As AI-driven imaging continues to evolve, its integration into routine cardiovascular risk assessment holds great promise for personalized medicine.
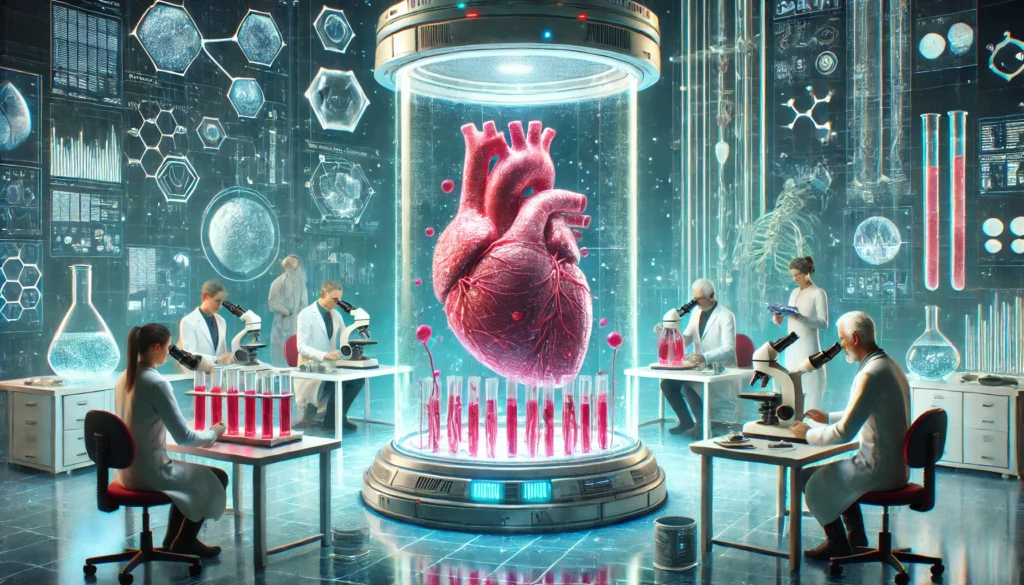
Emerging Biomarkers and Genetic Risk Factors
In addition to imaging advancements, the identification of novel biomarkers has significantly enhanced cardiovascular risk stratification. Traditional biomarkers such as cholesterol levels and blood pressure provide valuable information, but they do not capture the full spectrum of cardiovascular risk. Recent research has focused on inflammatory markers, genetic predispositions, and metabolic factors to refine risk assessment models.
One of the most studied biomarkers is high-sensitivity C-reactive protein (hs-CRP), which serves as an indicator of systemic inflammation. Elevated hs-CRP levels have been linked to an increased risk of cardiovascular events, making it a valuable addition to traditional risk models. Similarly, lipoprotein(a) [Lp(a)] has gained attention as an independent risk factor for atherosclerosis, particularly in individuals with a strong family history of CVD.
Genetic research has also uncovered significant insights into cardiovascular risk. Polygenic risk scores (PRS) aggregate the effects of multiple genetic variants to estimate an individual’s inherited predisposition to CVD. When combined with traditional risk factors, PRS provides a more comprehensive risk profile, enabling earlier interventions for high-risk individuals. As genetic testing becomes more accessible, its integration into routine cardiovascular risk assessment is expected to expand, paving the way for more personalized prevention strategies.
Digital Health and Wearable Technology in Cardiac Risk Prevention
The rise of digital health solutions and wearable technology has revolutionized cardiovascular risk prevention. Smartwatches and fitness trackers equipped with heart rate monitors, electrocardiogram (ECG) sensors, and oxygen saturation measurements provide real-time data on cardiovascular health. These devices enable continuous monitoring, allowing individuals and healthcare providers to detect early warning signs of cardiac abnormalities.
Beyond consumer wearables, medical-grade remote monitoring systems have gained traction in preventive cardiology. Devices capable of tracking blood pressure, heart rate variability, and atrial fibrillation episodes provide valuable data for risk assessment and intervention. Moreover, AI-driven analytics can identify patterns and trends in cardiovascular metrics, facilitating proactive healthcare management.
Telemedicine and mobile health applications have also played a crucial role in expanding access to preventive cardiovascular care. Virtual consultations, remote monitoring, and digital health coaching empower patients to take a proactive approach to heart health, reducing barriers to traditional healthcare services. As these technologies continue to evolve, their integration into cardiac risk stratification models will further enhance prevention strategies.
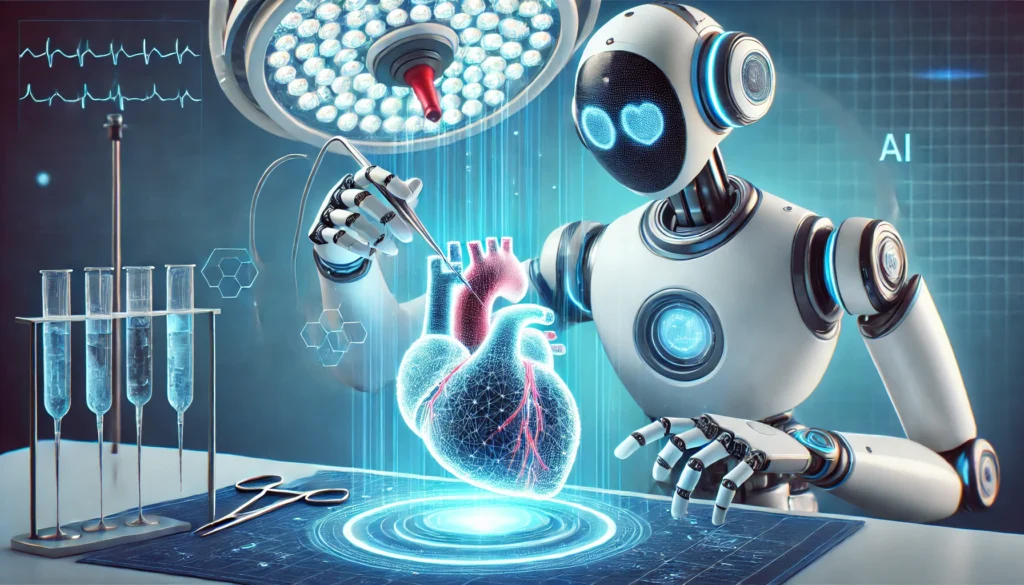
Frequently Asked Questions (FAQ) on Cardiovascular Risk Stratification
1. How has cardiovascular risk stratification evolved with recent technological advancements? Cardiovascular risk stratification has progressed significantly due to advancements in data analytics, machine learning, and personalized medicine. Traditional models primarily relied on broad demographic and lifestyle factors, but modern approaches integrate genetic profiling, advanced imaging techniques, and biomarker assessments to provide more precise risk evaluations. AI-powered tools can analyze vast datasets to detect early indicators of cardiovascular disease, offering tailored risk profiles for individuals. Additionally, wearable technology now enables continuous monitoring of heart health, providing real-time data that can refine cardiovascular risk stratification. As technology continues to evolve, risk assessment models will likely become even more individualized, improving early detection and preventive strategies.
2. What role does genetic testing play in refining cardiac risk stratification? Genetic testing has emerged as a powerful tool in cardiac risk stratification, particularly for identifying individuals with a predisposition to cardiovascular disease. Polygenic risk scores aggregate the effects of multiple genetic variations, allowing for a more accurate estimation of inherited risk. This method is especially beneficial for individuals with a family history of heart disease but no obvious traditional risk factors. Genetic insights can inform personalized prevention strategies, guiding lifestyle modifications and medical interventions tailored to an individual’s unique genetic profile. As research progresses, integrating genetic data with conventional cardiac risk index models will enhance the predictive accuracy of cardiovascular risk stratification.
3. How does the cardiac risk index compare to newer predictive models? The cardiac risk index has been a valuable tool in assessing perioperative and long-term cardiovascular risk, but newer predictive models incorporate additional factors to enhance precision. Traditional indices, such as the Revised Cardiac Risk Index (RCRI), focus on clinical variables like history of heart failure, kidney disease, and diabetes. However, emerging models integrate imaging modalities like coronary artery calcium scoring and biomarkers such as NT-proBNP, offering deeper insights into vascular health. These expanded approaches improve early risk detection, allowing clinicians to intervene before significant cardiac events occur. As predictive analytics continue to evolve, future iterations of the cardiac risk index will likely include even more sophisticated parameters.
4. Can artificial intelligence improve the accuracy of cardiovascular risk stratification? Yes, artificial intelligence (AI) is revolutionizing cardiovascular risk stratification by processing complex datasets with remarkable accuracy. AI-driven models can analyze imaging scans, genetic data, and electronic health records to identify subtle patterns that may be missed by traditional methods. Machine learning algorithms can refine cardiac risk stratification by continuously learning from new patient data, improving predictive accuracy over time. Furthermore, AI-powered chatbots and digital health assistants are helping patients understand their risk profiles and adhere to preventive measures. As AI technologies advance, they will play an increasingly integral role in optimizing cardiovascular risk assessment.
5. What are the limitations of current cardiac risk stratification methods? Despite significant advancements, current cardiac risk stratification models still have limitations. Many traditional models rely on population-based data, which may not accurately reflect an individual’s unique risk profile. Additionally, while biomarkers and genetic testing provide valuable insights, their accessibility and cost can be barriers for widespread implementation. Another challenge is the integration of multiple data sources—electronic health records, imaging results, and wearable device metrics—into a cohesive risk assessment framework. Efforts are ongoing to refine models by incorporating real-time data and improving standardization across healthcare systems. Future improvements in data integration and accessibility will enhance the precision and practicality of cardiovascular risk stratification.
6. How do social determinants of health influence cardiovascular risk stratification? Social determinants of health, including socioeconomic status, access to healthcare, and lifestyle factors, significantly impact cardiovascular risk stratification. Individuals from lower-income backgrounds may have limited access to preventive care, increasing their likelihood of undiagnosed or poorly managed cardiovascular risk factors. Environmental factors such as air pollution, neighborhood safety, and availability of healthy foods also play a crucial role in heart health. While traditional cardiac risk stratification models primarily focus on clinical and genetic factors, integrating social determinants into predictive algorithms could improve the accuracy of risk assessments. Addressing these broader influences is essential for a more holistic approach to cardiovascular disease prevention.
7. Can lifestyle interventions effectively modify cardiac risk index scores? Yes, lifestyle interventions can significantly impact cardiac risk index scores and overall cardiovascular health. Regular physical activity, a heart-healthy diet, and stress management are fundamental to reducing cardiovascular risk. Smoking cessation and moderation of alcohol intake are also critical in improving long-term outcomes. Emerging research suggests that individualized lifestyle plans, informed by genetic predispositions and metabolic responses, may offer the most effective risk reduction strategies. While medications can play a role in managing risk factors, long-term cardiovascular health is best achieved through sustainable lifestyle modifications tailored to an individual’s unique profile.
8. What emerging biomarkers are improving cardiovascular risk stratification? Emerging biomarkers are enhancing cardiovascular risk stratification by providing deeper insights into inflammation, metabolic health, and endothelial function. High-sensitivity C-reactive protein (hs-CRP) is widely recognized for its ability to detect chronic inflammation linked to cardiovascular disease. Lipoprotein(a) [Lp(a)] has also gained attention as an independent risk factor for atherosclerosis, particularly in individuals with a family history of heart disease. Additionally, newer markers such as trimethylamine-N-oxide (TMAO) and galectin-3 are being explored for their roles in predicting heart failure and vascular dysfunction. As research advances, these biomarkers will be increasingly integrated into routine cardiac risk index assessments, refining predictive models and guiding early intervention strategies.
9. How do wearable health devices contribute to cardiac risk stratification? Wearable health devices have transformed cardiac risk stratification by enabling continuous monitoring of heart rate, blood pressure, and other cardiovascular metrics. Devices equipped with electrocardiogram (ECG) sensors can detect arrhythmias, providing early warning signs of potential cardiac events. Smartwatches and fitness trackers offer real-time feedback on physical activity levels, helping users adopt heart-healthy behaviors. Beyond consumer wearables, medical-grade remote monitoring systems allow healthcare providers to track patient data remotely, enhancing early detection and intervention. As these technologies become more advanced, their integration into cardiovascular risk stratification models will further personalize risk assessment and prevention strategies.
10. What future innovations could further refine cardiovascular risk stratification? Future innovations in cardiovascular risk stratification will likely include greater integration of precision medicine, AI-driven analytics, and real-time physiological monitoring. Advances in multi-omics research, including genomics, proteomics, and metabolomics, will enable a more comprehensive understanding of individual cardiovascular risk profiles. AI-powered decision support systems will enhance clinical decision-making, offering personalized treatment recommendations based on vast datasets. Additionally, non-invasive imaging techniques will continue to improve, allowing for earlier detection of subclinical disease. As these innovations converge, the future of cardiac risk index assessment will be more dynamic, precise, and patient-centered, ultimately improving cardiovascular disease prevention and management.
Conclusion
Advancements in cardiovascular risk stratification have transformed the landscape of preventive cardiology, offering more precise and personalized approaches to risk assessment. The integration of novel biomarkers, advanced imaging techniques, and digital health solutions has significantly enhanced the accuracy of risk prediction models, enabling earlier interventions for high-risk individuals. As research continues to uncover new insights into cardiac risk factors, the future of cardiovascular prevention will rely on a multifaceted approach that combines genetic data, AI-driven analytics, and real-time monitoring technologies. By leveraging these innovations, healthcare providers can optimize cardiovascular risk stratification, ultimately improving patient outcomes and reducing the global burden of cardiovascular disease.
heart disease prevention, cardiovascular health assessment, cardiac wellness strategies, heart attack risk factors, advanced heart screening, predictive heart health, coronary artery disease prevention, cardiac biomarker analysis, AI in cardiology, genetic heart risk factors, cardiovascular disease detection, digital health monitoring, personalized heart care, heart disease diagnostics, inflammation and heart health, early heart disease signs, machine learning in cardiology, non-invasive cardiac tests, lifestyle and heart disease, wearable heart health technology
Further Reading:
Advancements in risk stratification and management strategies in primary cardiovascular prevention
Disclaimer
The information contained in this article is provided for general informational purposes only and is not intended to serve as medical, legal, or professional advice. While MedNewsPedia strives to present accurate, up-to-date, and reliable content, no warranty or guarantee, expressed or implied, is made regarding the completeness, accuracy, or adequacy of the information provided. Readers are strongly advised to seek the guidance of a qualified healthcare provider or other relevant professionals before acting on any information contained in this article. MedNewsPedia, its authors, editors, and contributors expressly disclaim any liability for any damages, losses, or consequences arising directly or indirectly from the use, interpretation, or reliance on any information presented herein. The views and opinions expressed in this article are those of the author(s) and do not necessarily reflect the official policies or positions of MedNewsPedia.