Understanding the Rise of Medical Artificial Intelligence
The last decade has ushered in a profound transformation in the healthcare industry, driven largely by rapid advancements in artificial intelligence. This revolution is not limited to research laboratories or tech startups—it is now deeply embedded in clinical practice. Medical artificial intelligence refers to the application of AI technologies, such as machine learning algorithms and natural language processing, to medical diagnosis, treatment planning, drug development, and patient care. Unlike previous innovations that focused solely on efficiency, today’s AI in medicine emphasizes personalization, precision, and predictive capabilities, reshaping the very fabric of healthcare delivery.
You may also like: How Top Digital Health Companies Are Revolutionizing Patient Care Through AI and Remote Monitoring
Across hospitals and clinics worldwide, AI systems are increasingly being deployed to sift through massive datasets—from electronic health records (EHRs) to radiological images—to uncover patterns that may escape even the most experienced human eye. These insights are not only accelerating the pace of diagnosis but are also enabling earlier detection of diseases, such as cancer or neurodegenerative disorders, often before symptoms appear. This proactive approach, empowered by artificial intelligence in medicine, is transforming the traditional model of reactive care into one that is anticipatory and data-driven.
As the global health ecosystem contends with challenges like rising costs, aging populations, and unequal access to medical services, the applications of artificial intelligence in medicine offer a promising solution. By automating repetitive tasks, optimizing resource allocation, and enhancing decision-making, AI technologies are supporting clinicians rather than replacing them. This collaborative model underscores the importance of developing AI tools that are transparent, explainable, and ethically sound—principles that form the foundation of trust in medical AI systems.
The integration of artificial intelligence into mainstream medicine is also supported by the growing body of research featured in peer-reviewed publications, such as the artificial intelligence in medicine journal. These publications provide critical validation for AI algorithms, highlighting their real-world effectiveness and limitations. By aligning innovations with clinical evidence, the medical community is forging a path toward responsible and effective AI adoption that enhances patient outcomes while safeguarding ethical standards.
Ultimately, the emergence of intelligence medicine represents a paradigm shift in how we understand health and treat disease. It is not just about smarter machines—it is about smarter medicine, informed by data, driven by compassion, and guided by human expertise.
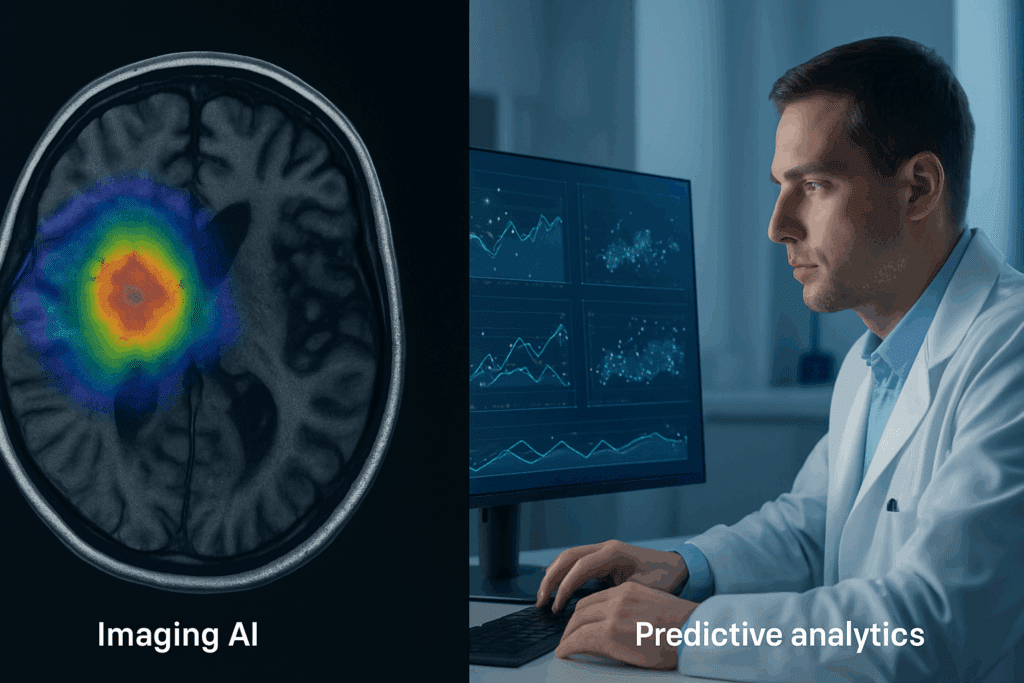
Redefining Diagnosis Through Predictive Analytics and Imaging AI
One of the most impactful contributions of AI in medicine is its ability to improve diagnostic accuracy through predictive analytics and advanced imaging technologies. Traditional diagnostic approaches often rely on human interpretation of symptoms, test results, and imaging data, which are inherently subject to variability and potential error. AI-based diagnostic tools, on the other hand, utilize deep learning models trained on vast datasets to recognize complex patterns and anomalies with high precision.
For instance, AI algorithms are now being used to detect early signs of diseases like diabetic retinopathy, breast cancer, and lung nodules in radiographic images with accuracy that rivals or even surpasses that of human radiologists. These AI systems not only provide faster results but also reduce the cognitive burden on physicians, allowing them to focus more on patient care. In this way, medical artificial intelligence serves as an indispensable assistant in the clinical workflow.
Beyond imaging, predictive analytics tools are increasingly integrated into electronic health record systems to flag patients at risk of developing specific conditions. For example, an AI model might analyze lab values, vital signs, and medication histories to predict the likelihood of sepsis or cardiac arrest in hospitalized patients. This predictive capability enables clinicians to intervene sooner, potentially saving lives and reducing hospital readmissions.
Importantly, these diagnostic advancements are not just theoretical. Studies published in the artificial intelligence in medicine journal have documented real-world implementations of AI tools in clinical settings, showcasing improved diagnostic efficiency and better patient outcomes. These outcomes reinforce the value of AI medical technology and encourage further investment in its development.
Despite the promising results, challenges remain. AI systems must be rigorously validated to avoid biases that could disproportionately affect marginalized populations. Additionally, regulatory bodies like the FDA are working to establish clear guidelines for evaluating the safety and efficacy of AI-based diagnostic tools. These measures are critical to ensure that the adoption of AI in diagnostic medicine aligns with principles of fairness, accuracy, and transparency.
As technology continues to evolve, the role of artificial intelligence in medicine will only grow more central to the diagnostic process. Through continued research, collaboration, and ethical oversight, AI stands poised to redefine the art and science of diagnosis for the better.
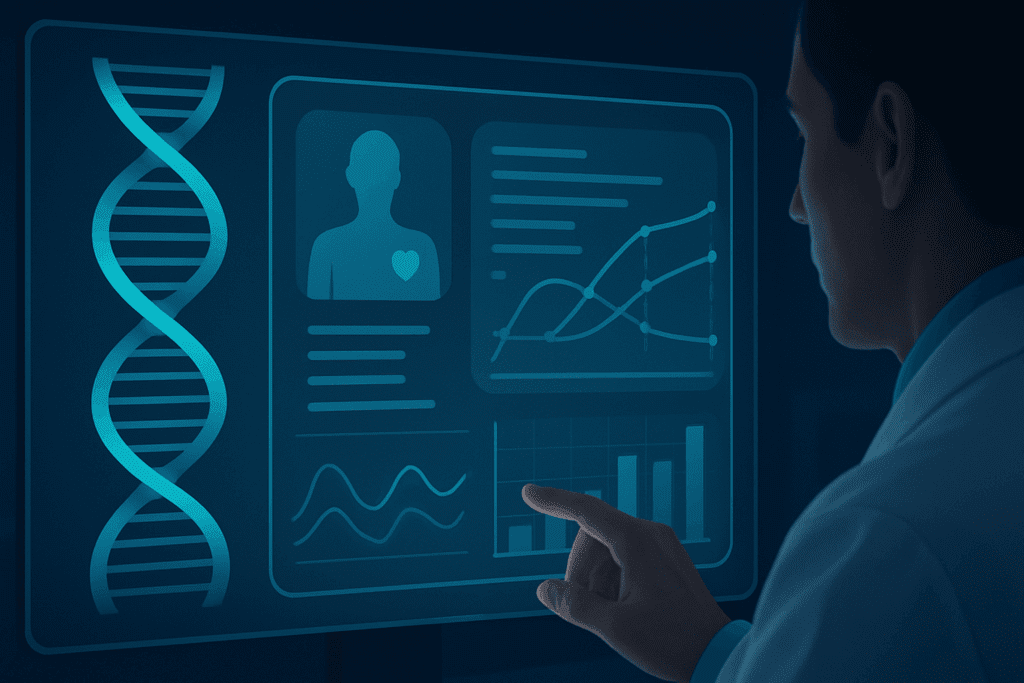
AI-Powered Treatment Planning and Personalized Medicine
Beyond diagnosis, artificial intelligence is playing an increasingly vital role in treatment planning and the broader shift toward personalized medicine. Traditional treatment protocols often follow standardized guidelines based on population averages, which may not account for individual patient differences in genetics, lifestyle, or comorbidities. AI enables a more nuanced approach by analyzing complex datasets to tailor treatments to the unique characteristics of each patient.
For instance, oncologists are now leveraging AI to design personalized cancer therapies by integrating genomic data, tumor histology, and previous treatment responses. Machine learning models can predict how a patient might respond to specific chemotherapy drugs or immunotherapies, thereby minimizing trial-and-error approaches and improving therapeutic efficacy. This marks a significant advancement in intelligence medicine, where data-driven insights are used to guide clinical decisions with greater precision.
Another area where AI medical technology is proving transformative is in chronic disease management. For patients with conditions like diabetes or hypertension, AI-powered mobile applications and wearables continuously monitor vital signs and behavioral patterns. These tools can alert both patients and providers to deviations that may warrant medical intervention, enabling proactive and personalized care.
Treatment AI tools also facilitate medication optimization by identifying potential drug interactions, adverse effects, and dosage recommendations based on a patient’s complete medical profile. This level of personalization not only enhances safety but also boosts adherence, as patients receive therapies that are more tolerable and effective. Furthermore, AI’s ability to assimilate new data in real time ensures that treatment plans evolve as patient needs change.
The development of these sophisticated tools is frequently documented in peer-reviewed sources such as the artificial intelligence in medicine journal, lending scientific credibility to their use in clinical settings. These publications often feature case studies and clinical trials that demonstrate the practical benefits and limitations of AI-assisted treatment planning.
However, the transition to AI-supported personalized medicine is not without its hurdles. Issues of data privacy, interoperability between systems, and the need for clinician training must be addressed to fully realize the benefits of treatment AI. Nevertheless, the trajectory is clear: artificial intelligence is becoming a cornerstone of individualized care, helping healthcare providers move from reactive treatment to proactive health management.
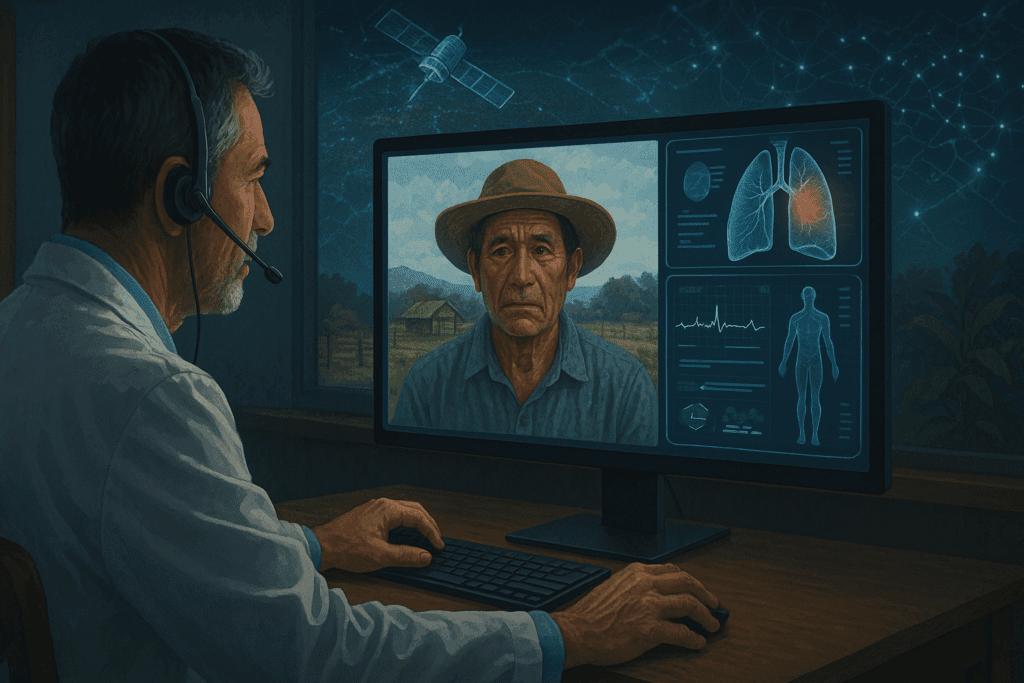
Transforming Patient Outcomes and Healthcare Delivery
Perhaps the most compelling justification for integrating AI in medicine is its demonstrable impact on patient outcomes. Improved diagnostic accuracy, timely interventions, and tailored treatments converge to create a more responsive and effective healthcare system. Patients benefit not only from earlier detection and intervention but also from reduced hospital stays, fewer complications, and improved quality of life.
One particularly promising application of AI is in postoperative care and rehabilitation. By using data from wearable sensors and patient-reported outcomes, AI systems can monitor recovery progress and identify signs of complications before they escalate. This approach not only enhances patient safety but also reduces the burden on healthcare providers by enabling remote monitoring and follow-up.
Moreover, AI technologies support healthcare delivery in underserved or rural areas where medical expertise may be limited. Telemedicine platforms enhanced with AI tools can assist general practitioners in making specialist-level decisions, thereby democratizing access to high-quality care. This is especially significant in global health contexts where resource constraints demand innovative solutions.
AI advancements in healthcare also extend to hospital operations and administrative processes. Predictive models can forecast patient admissions, optimize staffing schedules, and manage supply chains, leading to more efficient resource utilization. These operational improvements indirectly benefit patients by reducing wait times and ensuring timely access to care.
The cumulative effect of these innovations is a shift toward a value-based care model, where outcomes and patient satisfaction are prioritized over volume. As intelligence medicine continues to mature, we can expect to see more data-driven metrics being used to assess clinical effectiveness and inform policy decisions. By aligning incentives with outcomes, AI-powered systems are paving the way for a more sustainable and patient-centered healthcare ecosystem.
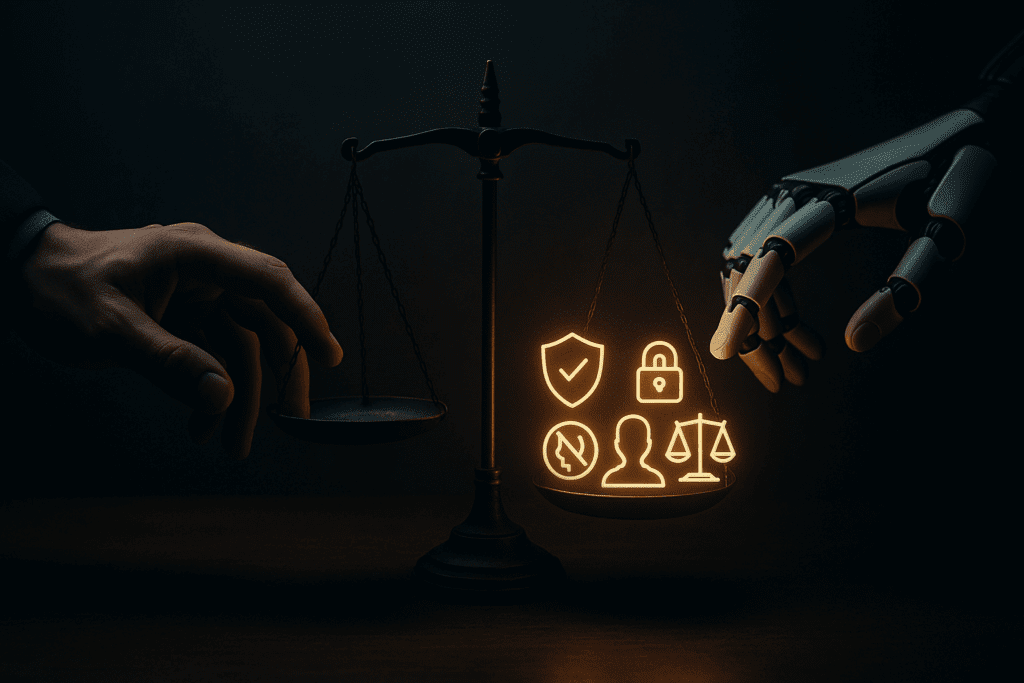
Ethical Considerations and the Role of Human Oversight
Despite its potential, the integration of artificial intelligence in medicine raises important ethical questions that must be addressed to maintain public trust and uphold professional standards. One of the foremost concerns is data privacy. The success of AI systems depends on access to vast amounts of patient data, which must be collected, stored, and analyzed in ways that respect patient autonomy and confidentiality.
To mitigate these concerns, healthcare organizations are adopting robust data governance frameworks that emphasize transparency, informed consent, and security. Advanced encryption methods and decentralized data storage are also being explored to enhance data protection. These efforts are essential to ensuring that AI medical technology does not compromise the rights of patients in pursuit of innovation.
Another critical issue is algorithmic bias. If the data used to train AI systems is skewed or unrepresentative, the resulting models may perpetuate or even exacerbate health disparities. For instance, a diagnostic algorithm trained predominantly on data from one ethnic group may perform poorly when applied to other populations. Addressing this requires not only technical adjustments but also a commitment to diversity and inclusion in data collection and model validation.
The definition of AI in medicine must also include the principle of human oversight. AI should augment, not replace, clinical judgment. Physicians and other healthcare professionals must remain the ultimate decision-makers, using AI tools as supportive resources rather than definitive authorities. This collaborative model ensures that ethical and contextual considerations are factored into each medical decision.
Furthermore, the medical community is increasingly calling for the establishment of ethical review boards specifically focused on AI applications. These boards can provide guidance on responsible innovation, assess risks and benefits, and ensure compliance with evolving regulations. Such oversight is vital for fostering an environment where innovation thrives without compromising ethical integrity.
By proactively addressing these ethical dimensions, we can harness the full potential of medical artificial intelligence while upholding the core values of medicine: compassion, trust, and respect for human dignity.
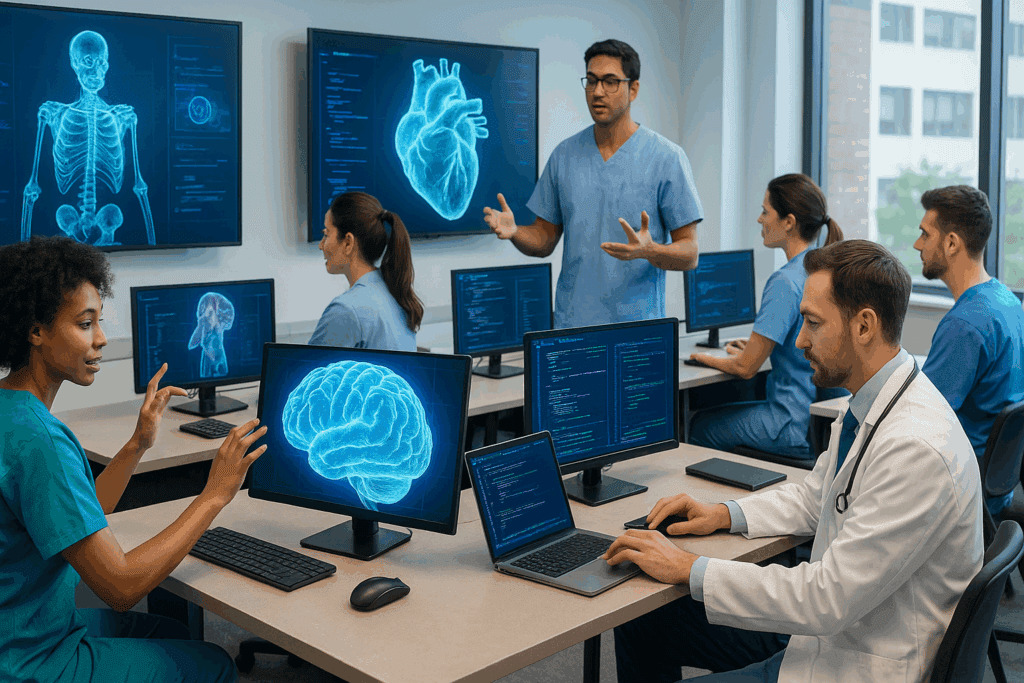
AI Education and the Evolving Role of the Healthcare Professional
As AI becomes more deeply embedded in clinical practice, the role of the healthcare professional is also undergoing transformation. Rather than being sidelined by automation, clinicians are being empowered with new tools that enhance their capabilities and expand their roles. To fully leverage the benefits of AI, however, healthcare providers must be equipped with the knowledge and skills necessary to interpret and apply AI-generated insights responsibly.
Medical education is beginning to evolve in response to this need. Leading medical schools and residency programs are integrating coursework on data science, machine learning, and AI ethics into their curricula. These programs aim to create a new generation of clinicians who are not only proficient in their respective specialties but also fluent in the language of AI medical technology.
Continuing education is equally important for practicing professionals. Workshops, certifications, and online learning platforms are helping clinicians stay current with the latest AI advancements in healthcare. These educational efforts ensure that AI tools are used appropriately and effectively, reducing the risk of misuse or overreliance.
The shift also brings new interdisciplinary collaboration. Physicians, data scientists, software engineers, and ethicists must work together to design, implement, and evaluate AI systems. This collaborative model enriches the development process and ensures that tools are clinically relevant, ethically sound, and user-friendly.
Ultimately, the future of intelligence medicine depends not only on technological progress but also on human adaptability. By fostering a culture of lifelong learning and cross-disciplinary cooperation, the medical community can ensure that AI becomes a trusted partner in care, enhancing rather than diminishing the human touch that lies at the heart of healing.
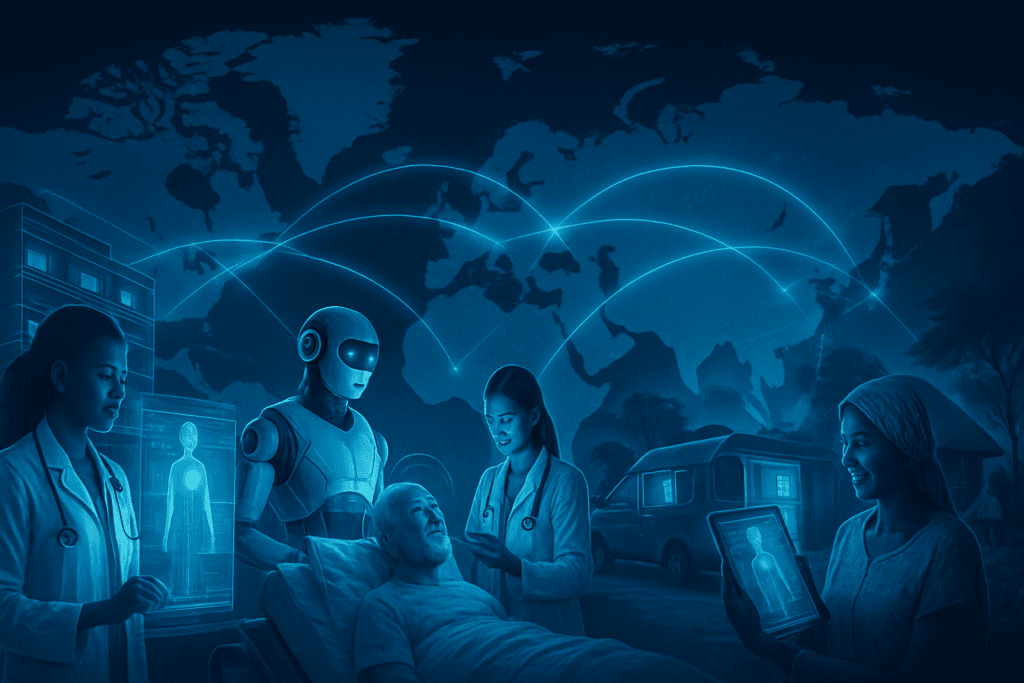
Looking Ahead: The Future of AI in Medicine and Its Impact on Global Health
The road ahead for AI in medicine is one of immense opportunity and ongoing evolution. As algorithms become more sophisticated and datasets more comprehensive, we can expect AI tools to play an even greater role in clinical decision-making, public health surveillance, and global disease management. The convergence of AI with other technologies such as genomics, robotics, and telemedicine will further amplify its impact, creating integrated ecosystems of care that are both scalable and sustainable.
In low- and middle-income countries, the deployment of AI medical technology offers a chance to leapfrog traditional barriers to healthcare access. Mobile-based AI diagnostic tools, for instance, can bring critical services to remote areas, reducing reliance on centralized infrastructure. Such innovations can help bridge the gap between urban and rural health systems, promoting equity and improving population health outcomes.
Moreover, the continued refinement of AI medical definitions and regulatory frameworks will enhance public understanding and confidence. Clear definitions and transparent communication about the capabilities and limitations of AI are essential for informed consent and shared decision-making. As the artificial intelligence in medicine journal and similar publications continue to disseminate evidence-based insights, both professionals and the public will be better equipped to navigate this new frontier.
It is also likely that new AI-driven fields will emerge, such as predictive public health modeling or AI-assisted mental health interventions. These applications will require rigorous evaluation and ethical oversight, but their potential to address unmet needs is substantial. As we explore these frontiers, the importance of maintaining a patient-centered approach cannot be overstated.
In embracing the possibilities of medical artificial intelligence, we must remain grounded in the values that define good medicine: empathy, integrity, and a commitment to health for all. AI is not a replacement for the physician’s intuition or the nurse’s compassion—it is a tool that, when used wisely, can magnify the best of what human care has to offer.
Frequently Asked Questions: AI in Modern Medicine
1. What are the lesser-known challenges of integrating medical artificial intelligence into clinical practice?
While much attention is given to the benefits of medical artificial intelligence, several nuanced challenges often go unnoticed. One key issue is the adaptation gap between AI developers and clinicians—many AI tools are built without sufficient input from medical professionals, leading to misalignment with actual clinical workflows. Moreover, the frequent updates and iterations in AI models can create confusion among practitioners regarding which version they are using and how its logic has changed. Institutional resistance to change and legacy infrastructure limitations also slow adoption, as integrating AI medical technology often requires overhauling existing systems. Lastly, the cultural shift needed to embrace AI in medicine—moving from experience-driven to data-driven decision-making—can create tensions within care teams, especially when trust in automation is still evolving.
2. How are AI technologies being applied in low-resource healthcare environments?
In low-resource settings, the applications of artificial intelligence in medicine are expanding in practical and highly impactful ways. AI-powered diagnostic tools, which operate on mobile devices, can assist community health workers in identifying diseases such as tuberculosis or malaria without the need for on-site labs. These tools not only reduce diagnostic turnaround time but also provide a cost-effective alternative to traditional testing. Additionally, AI in medicine is being used for predictive modeling to allocate limited medical supplies, such as vaccines or antibiotics, more efficiently. AI chatbots and decision-support systems can guide frontline healthcare providers in treatment protocols when specialists are not available. This broader accessibility showcases how AI medical technology is helping bridge gaps in global health equity, especially in rural or underserved communities.
3. How does AI influence mental health treatment and diagnosis?
The integration of artificial intelligence in medicine is bringing about significant progress in mental health care. By analyzing speech patterns, facial expressions, and even keystroke dynamics, AI can identify early signs of depression, anxiety, and other psychiatric disorders with a level of objectivity that traditional assessments sometimes lack. AI-powered platforms also enable continuous monitoring of patients through wearables or apps, offering timely alerts when symptoms escalate. Additionally, chatbots trained on cognitive behavioral therapy frameworks are providing accessible, low-barrier mental health support. These AI advancements in healthcare are not meant to replace human therapists but to augment mental health services in scalable ways. In the future, we may see AI applications extending into personalized treatment pathways based on behavioral and biometric data.
4. What are some ethical implications of using treatment AI for life-or-death decisions?
Using treatment AI in critical care settings introduces complex ethical dilemmas, especially when algorithms suggest decisions that affect survival. For example, in intensive care units, AI models may prioritize patients based on probability of recovery, raising questions about fairness, bias, and transparency. There is concern that such systems could inadvertently replicate systemic inequities present in training data, particularly if they undervalue minority populations or those with complex health histories. Additionally, reliance on treatment AI must be carefully managed to ensure clinicians retain ultimate authority, blending machine-generated insights with human compassion and contextual judgment. These concerns are increasingly discussed in the artificial intelligence in medicine journal, which calls for frameworks to ensure that AI use in high-stakes environments aligns with ethical, legal, and societal expectations.
5. How does the ai medical definition differ across disciplines?
The ai medical definition can vary considerably depending on the discipline in which it is applied. In radiology, for example, it often refers to algorithms trained to detect patterns in imaging data, whereas in pharmacology, it may describe systems that model drug interactions and predict efficacy. In surgery, it can encompass robotic systems guided by AI for precision and minimal invasiveness. The broadness of this definition reflects the versatility of AI medical technology, but also poses challenges for standardization and regulation. This diversity underscores the importance of multidisciplinary collaboration to ensure consistent understanding and application of artificial intelligence in medicine across different healthcare domains.
6. Can artificial intelligence be trained to detect rare or emerging diseases?
Yes, one of the most promising frontiers in medical artificial intelligence is its capacity to detect rare or newly emerging diseases. Through techniques like anomaly detection and unsupervised learning, AI systems can flag cases that deviate from known patterns, prompting further clinical investigation. When coupled with global health surveillance data, these models can even assist in identifying outbreak hotspots before they spread widely. AI advancements in healthcare are making it feasible to recognize subtle biomarkers or combinations of symptoms that would otherwise be dismissed as noise. With proper training data and validation, these models can become critical components of early-warning systems for conditions ranging from genetic disorders to novel pathogens.
7. What role does the artificial intelligence in medicine journal play in advancing the field?
The artificial intelligence in medicine journal is a foundational resource for professionals, researchers, and policymakers seeking to stay informed about the latest developments in AI healthcare integration. It serves as a platform for peer-reviewed research, case studies, and critical reviews that assess both the potential and limitations of current technologies. Beyond publishing new discoveries, the journal also influences regulatory decisions by disseminating best practices and risk assessments. Its interdisciplinary focus ensures that submissions cover not just technical aspects, but also ethical, legal, and social implications of intelligence medicine. By fostering rigorous scientific dialogue, the journal helps maintain the credibility and responsible growth of this rapidly evolving field.
8. How are AI systems evaluated for safety and reliability in clinical environments?
Before AI systems are deployed in clinical practice, they undergo a series of evaluations designed to assess safety, reliability, and clinical utility. These assessments often involve retrospective validation on historical datasets, followed by prospective trials in real-world settings. Regulatory bodies such as the FDA require extensive documentation demonstrating that an AI medical technology can perform consistently across diverse patient populations. Furthermore, ongoing post-market surveillance ensures that any deviations or failures are promptly identified and addressed. The applications of artificial intelligence in medicine are thus governed by a blend of technical scrutiny and regulatory oversight, emphasizing patient safety and transparency at every stage.
9. In what ways are AI medical terms shaping public perception and understanding?
AI medical terms—such as “machine learning-based diagnosis” or “algorithmic triage”—are increasingly entering public discourse, influencing how patients perceive healthcare technologies. While these terms convey innovation, they can also create confusion or unrealistic expectations when not properly explained. For instance, patients might assume that AI guarantees accuracy or objectivity, overlooking the human oversight still required for safe use. Media representations of AI often amplify its capabilities without addressing limitations, making public education a key priority. Healthcare providers must take the lead in demystifying these terms and communicating clearly about how artificial intelligence in medicine supports, rather than replaces, human care.
10. What are the long-term implications of intelligence medicine for the healthcare workforce?
Over the long term, intelligence medicine is poised to reshape the structure and dynamics of the healthcare workforce. Roles that involve repetitive or administrative tasks may be partially automated, allowing clinicians to focus more on patient interaction and complex decision-making. Simultaneously, new positions will emerge at the intersection of healthcare and data science, including AI system analysts, ethics officers, and clinical AI trainers. The workforce will require continuous reskilling to keep pace with evolving tools and standards. Additionally, interdisciplinary collaboration will become the norm, as teams comprising physicians, engineers, and data scientists work together to design and deploy effective solutions. Far from eliminating jobs, AI in medicine is likely to enrich the workforce by fostering greater specialization and collaboration.
Conclusion: Embracing the Promise of Artificial Intelligence in Medicine
Artificial intelligence is no longer a distant promise or a futuristic ideal—it is a present-day reality reshaping every facet of modern healthcare. From enhancing diagnostic precision to personalizing treatment plans and transforming patient outcomes, the applications of artificial intelligence in medicine are as diverse as they are impactful. These tools offer new hope for more efficient, equitable, and effective healthcare delivery, bridging gaps that once seemed insurmountable.
Yet with this promise comes responsibility. The integration of AI medical technology demands rigorous attention to ethics, data governance, and professional oversight. It challenges us to rethink medical education, redefine roles, and reimagine care delivery with patients at the center. It also compels us to advocate for inclusivity and fairness in algorithm design, ensuring that no one is left behind in the pursuit of innovation.
As research continues to evolve—reflected in the ongoing contributions of the artificial intelligence in medicine journal and other scientific forums—we gain clearer insight into what works, what needs refining, and where the greatest opportunities lie. By embracing a balanced, evidence-based approach to implementation, we can unlock the full potential of AI without compromising our core values.
In the end, artificial intelligence in medicine is not merely about smarter systems. It is about forging a smarter, more compassionate healthcare future—one where technology empowers clinicians, elevates patients, and strengthens the human connection that lies at the heart of healing.
Was this article helpful? Don’t let it stop with you. Share it right now with someone who needs to see it—whether it’s a friend, a colleague, or your whole network. And if staying ahead on this topic matters to you, subscribe to this publication for the most up-to-date information. You’ll get the latest insights delivered straight to you—no searching, no missing out.
Further Reading:
Artificial Intelligence in Healthcare: Revolutionising Diagnosis and Treatment
Revolutionizing healthcare: the role of artificial intelligence in clinical practice
Artificial intelligence in healthcare: transforming the practice of medicine